Many AI-Guided Psychosis Prediction Tools Not Much Better Than Chance
Abstract
While a series of machine learning programs did well when trained on a particular set of patient data, their ability to predict outcomes in independent samples was not impressive.
Are machine learning programs that try to predict which patients will respond to a certain medication the next big thing in psychiatry? A recent report in Science suggests that at least for models predicting antipsychotic response, the answer is not quite yet.
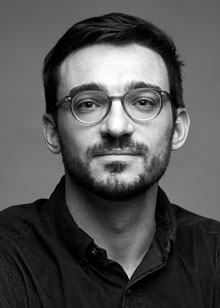
Adam Chekroud, Ph.D., said that clinical prediction models built off small samples generated more enthusiasm than warranted due to their novel nature.
Taking advantage of a large repository of schizophrenia clinical trial data, researchers at Yale University and colleagues developed and trained machine learning models for antipsychotic response. While all the models appeared promising in training, when they tried to predict patient response in an independent sample, none performed better than chance.
“The take-home message is that a lot of these models are good at predicting the past, but that’s not the same as predicting the future,” said lead investigator Adam Chekroud, Ph.D., the president and co-founder of Spring Health and an assistant adjunct professor at Yale University. Spring Health is a behavioral health startup that uses precision medicine tools to match patients with the appropriate treatment plan.
Chekroud and colleagues made use of the Yale Open Data Access (YODA) Project, an archive of data from over 200 clinical trials. From this extensive list, they selected five trials that compared the effects of antipsychotics versus placebo. The five were chosen because they used nearly the same data collection procedures and outcome measures but enrolled different sets of patients; for example, one trial involved older adults, while another involved young adults with first-episode psychosis. Together, there were 1,513 patients with psychosis in the five trials.
The researchers next developed a learning algorithm that examined over 200 demographic and clinical variables to identify patterns that could predict which patients would respond or remit after four weeks of antipsychotic treatment. The researchers trained this algorithm separately on each of the five trials; for each trial they also tested four established definitions of remission or response (for example a 50% reduction in a patient’s Positive and Negative Syndrome Scale score to mark treatment response).
As expected, the algorithm was very good at studying the data in its clinical population and finding patterns that predicted which patients improved with treatment; accuracy rates ranged from 66% to 77%. However, when each trained algorithm was tasked to take what it had learned and predict outcomes in one of the other four clinical trials, the program failed to break 50%, no matter which definition of response or remission was used.
“This drop-off suggests that these prediction programs are just ‘learning’ certain idiosyncrasies within their small dataset,” rather than a true set of clinical parameters associated with treatment response, Chekroud said.
As a next step, the research team increased the testing sample by training a program on four of the trials, then testing the program on the fifth. With this approach, the resulting algorithms were still only about 54% accurate at predicting treatment response, no matter which four trials were used for testing.
Chekroud told Psychiatric News that these poor prediction results are not an indictment on AI-guided prediction tools. “My Ph.D. dissertation was on this topic; I want these models to succeed,” he said.
The problem is that prediction studies built off small sample analysis generated more enthusiasm than perhaps warranted, he continued. “I think small sample studies with prediction models are much like phase 1 drug trials,” he explained. Clinicians don’t get too excited about the latter because they know many promising drug candidates will fizz out by phase 2 or 3. AI is novel, Chekroud said, but the same expectations should apply.
So how can these tools be improved? “There is more and more recognition among the community of data scientists that to get the right output, we need the right data going in,” said Joshua Gordon, M.D., Ph.D., the outgoing director of the National Institute of Mental Health. “Clinical data are the foundation, but there are missing pieces we need to add on top.”
Some researchers believe that given the multifactorial causes of schizophrenia, adding genetic and/or brain imaging data to a patient’s clinical history will improve accuracy, though Chekroud is not convinced. “I’m not sure genetic and imaging data are scalable to the levels we need for prediction. Also, adding these elements really changes the complexity and cost of these programs.”
An alternative to focusing on what the brain looks like or its DNA composition is to track what the brain is doing, Gordon suggested. “The brain performs specific cognitive and behavioral tasks [that] can be divided into categories,” he said. These categories, or constructs, include functions like perception or reward processing. Gordon believes researchers can devise inexpensive computer-based tasks that target a particular construct and provide an objective measure of how it is performing.
“If we can make these behaviors computationally describable, it gives us something to add to these decision-making tools to make them more precise,” Gordon said. He noted that NIMH approved the first set of research awards for this large initiative, termed IMPACT-MH, last year.
“We haven’t achieved our goal of precision psychiatry yet, but these predictive programs are getting incrementally better,” Chekroud said. It may not be flashy, “but this is what good science looks like.”
The study authors reported no external funding for this research. ■