Can Machine Learning Decode Depression?
Abstract
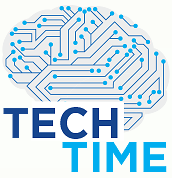
I think of depression, or more specifically major depressive disorder (MDD), as an umbrella term that encompasses many biologically different, although somewhat overlapping, conditions. These different types of MDD have sometimes been referred to as subtypes and categorized differently, a longstanding point of discussion within psychiatry over the last 100 years. Many different risk factors have been identified for depression, and choosing the right depression treatment can be tricky, as it remains difficult to predict how a person will respond to a particular medication or to psychotherapy. Given this background, can data science, and in particular machine learning, help revolutionize how we diagnose, treat, and monitor depression? A growing line of evidence gives us hope.
Machine learning was originally developed through the study of artificial intelligence, defined as computers that have the ability to learn without being explicitly programmed. Machine learning recognizes patterns in data that humans cannot detect either visually or by traditional analytic techniques. Machine learning requires machines to do just that, to learn. While it can take some time for machines to be “trained” on data and for useful algorithms to arise, once this has happened, machines excel at data analysis. Machine learning tools have the ability to speedily process huge amounts of data, with none of the fatigue that humans would rapidly face.
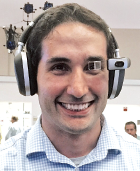
The combination of machine learning and big data is a perfect match and has huge potential in health care and mental health care. While it may sound like science fiction, machine learning is already all around us. Some examples are voice recognition, fraud detection, and spam detectors. In our own research at Brain Power, we are already witnessing how big data and machine learning can help us to bridge the gaps between educational, health, and social services for children and adults with autism.
Can machine learning predict the extent and length of someone’s depression, right from the outset? In a study published in the January 5 Molecular Psychiatry, baseline data from over a thousand people with MDD were analyzed. The aim was to predict the severity and chronicity of the subjects’ depression. The authors compared the use of traditional analytics and machine learning approach. They found that machine learning could not only help predict the characteristics of a person’s depression, but also do this more effectively, and with less information, than traditional approaches. The authors concluded that machine learning could be a clinically useful way to stratify depression.
Machine learning can also be used to link clinical depression with biomarkers. In a study published in the February 5 PLoS One, machine learning tools were used in addition to traditional statistics to analyze the relationship between 67 biomarkers in 5,227 research subjects. This hybrid technique was able to identify three biomarkers for depression, namely red cell distribution of width, serum glucose, and total bilirubin. Red cell distribution of width has been linked to inflammation in the body, and a line of research has linked depression and inflammation. Serum glucose tests are used to screen for diabetes, a condition that has a complex relationship with depression. Lastly, it has been suggested that bilirubin is an antioxidant, and depression has been linked to oxidative stress. While some of these associations are tenuous, or speculative at best, this study does identify potential biomarkers that can be taken forward into the next round of research.
One of the most difficult jobs for psychiatrists is to assess suicide risk in our patients. A particular challenge is incorporating all of the clinical data that we obtain, such as patients’ history of depression, their use of drugs, and their past suicide attempts, into producing a suicide risk assessment. What if we had a tool that could incorporate all of the clinical data we obtain about a patient in a more quantitative way, helping us to more accurately identify high-risk patients and provide preventive care? A pilot study in the March 15 Journal of Affective Disorders, using data from 144 patients with mood disorders, was able to produce three machine learning algorithms that could distinguish between people who had attempted suicide and those who had not based on each patient’s prior clinical data. The prediction accuracy varied between 65 to 72 percent.
One central challenge is being able to predict how patients will respond to psychiatric treatments. Some companies have suggested that genetic testing could help with prediction. A study of 4,041 patients with depression published in the January 20 Lancet Psychiatry found that by using a machine learning approach, patients’ response to citalopram could be predicted with a highly statistically significant accuracy of 64.6 percent.
In the near term, humans will continue to make the majority of psychiatric diagnoses and provide treatment. Technological approaches, such as machine learning, but also including telemedicine, smartphone apps, and wearable devices, have the potential to expand access and augment the work of clinicians. While the research noted here is still preliminary, it illustrates the promise of data science, and more specifically machine learning, in mental health. ■
Information on Brain Power can be accessed here. An abstract of “Testing a Machine-Learning Algorithm to Predict the Persistence and Severity of Major Depressive Disorder From Baseline Self-Reports” is available here. An abstract of “Identifying a Clinical Signature of Suicidality Among Patients With Mood Disorders: A Pilot Study Using a Machine Learning Approach” is located here. An abstract of “Cross-Trial Prediction of Treatment Outcome in Depression: A Machine Learning Approach” can be accessed here.