Could EEG Help Predict Antidepressant Response?
Abstract
Researchers are turning to machine learning programs to see if brain activity patterns can offer some insight into patients most likely to respond to certain antidepressants.
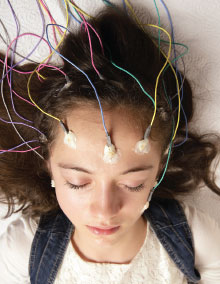
Electroencephalography (EEG)—which detects electrical activity in the brain—may hold promise as a tool to predict how likely a patient with depression is to respond to specific treatments. New research is using machine learning software to unlock the secrets hidden within the oscillating waves of an EEG recording.
“In the past, EEG measurements were commonly used in research to assess brain activity and health,” said Faranak Farzan, Ph.D., the Chair in Technology Innovation for Youth Addiction Recovery and Mental Health at Simon Fraser University in Canada. “But EEGs lost their glory when new brain imaging tools like functional MRI [fMRI] came out.”
Advances in technology are now helping EEGs make a comeback, Farzan said. “As with other technologies, EEGs have become less expensive and more portable, making them more practical in research,” she explained.
The increased accessibility of EEG machines has helped fuel a resurgence in studies looking for depression biomarkers in EEG readings. “EEG readouts provide a direct measurement of brain electrical activity,” noted Amit Etkin, M.D., Ph.D., a professor of psychiatry and behavioral sciences at Stanford University. “fMRI measures blood flow, which is only an indirect measurement of brain activity.”
Still, several hurdles remain before EEG can be used as a diagnostic for patients with depression. A 2018 analysis by the APA Task Force on Novel Biomarkers and Treatments noted that numerous small studies have reported that certain EEG readings can predict treatment response, but they have yet to be replicated in other studies. These studies have also used different machines and measured different types of brainwaves, providing very little consistency in the protocols.
That’s where technology can help again, Farzan told Psychiatric News. “With today’s computing power, we can look at EEG signals much more comprehensively than before.” Rather than focusing on just one or two specific types of brainwaves, researchers can compile all the different frequencies recorded by EEGs and construct maps of brain connectivity. Machine learning software—algorithms that scan large amounts of data to find patterns—then analyzes these connectivity profiles to look for signals that distinguish patients who respond to a treatment from those that do not.
Etkin recently led a study that tested machine learning software on EEG readings from 221 adults with depression who participated in a clinical trial known as EMBARC (Establishing Moderators and Biosignatures of Antidepressant Response in Clinic Care). The trial involved patients with depression who were randomized to eight weeks of sertraline or placebo. At the start of the trial, the researchers collected brain scans, EEGs, behavioral tests, and DNA samples from the study participants.
His analysis identified a specific set of EEG signals between the parietal and occipital lobes of the brain that were more active among patients who responded to sertraline relative to those who did not. What’s more, when Etkin and his team tested their software on a separate set of EEG readings from 152 patients who were part of a clinical study of transcranial magnetic stimulation (TMS) for depression, they found the opposite correlation with this parietal-occipital signal. Patients who had EEG signals indicative of a poor sertraline response were more likely to respond to TMS.
“This finding was especially promising from a clinical care standpoint,” said Etkin, who recently took a leave from Stanford to launch an EEG biomarker company called Alto Neuroscience. “We could potentially use EEG to guide treatment selection for patients.”
Farzan has also been using machine learning to scour EEG readings as part of a study similar to EMBARC known as the Canadian Biomarker Integration Network in Depression (CAN-BIND). This multisite study enrolled 180 adults with depression and looked for biomarkers that might predict response to escitalopram. As with Etkin’s study, Farzan’s team was able to find baseline EEG patterns that predicted patients who would respond well to escitalopram. Her analysis also showed that several brainwaves that change in response to escitalopram are also affected by electroconvulsive therapy (ECT), suggesting there are overlaps in how antidepressants and ECT operate.
Alik Widge, M.D., Ph.D., an assistant professor of psychiatry at the University of Minnesota and member of the Task Force on Novel Biomarkers and Treatments, said that clinical use of EEG to predict treatment response in patients with depression is likely still years away, but these results are still an important step forward in the field.
“This research is an example of how to build a good a set of data and how to analyze that data,” Widge told Psychiatric News.
Widge noted that the sample sizes of these studies are several times larger than previously published EEG biomarker research and included a more diverse group of patients from multiple sites. “To keep moving this tool toward clinical use, we need to have EEG data out there on patients who are representative of real-world practice and for a variety of drugs,” he said.
“The challenge is that these studies still enroll patients based on the criteria of DSM-5 major depression,” Widge continued. “Depression is such a heterogenous disorder that it will take a lot of data before we can accurately separate the signal from the noise.”
Etkin understands that it is likely to be years before EEG for targeting antidepressant treatment is a regular part of clinical practice, but he thinks his findings provide a hopeful message on treating depression. “Ever since Irving Kirsch’s 2008 paper suggested that antidepressants are no better than placebo pills, many people have expressed the sentiment that we shouldn’t be using these drugs at all,” he said. “Our findings, and other biomarker research, show that these drugs are very effective in the right patients.”
EMBARC was supported by multiple grants from the National Institutes of Health. CAN-BIND is supported by the Ontario Brain Institute, with additional funding provided by the Canadian Institutes of Health Research, Lundbeck, Bristol-Myers Squibb, Pfizer, and Servier. ■