Software Aims to Predict Patients Likely to Stop Antidepressants
Abstract
Such information may be able to help doctors identify the patients who need additional support to remain in treatment.
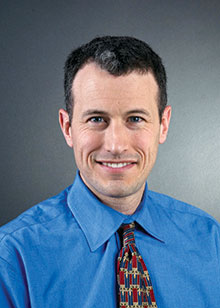
Roy Perlis, M.D., says that software that predicts the risk of patients discontinuing their medication could help physicians triage patients to ensure optimal medication adherence.
Much of the attention around precision medicine for major depression has centered on finding the most effective antidepressant for each individual patient. But even an optimal medication will not work if the patient doesn’t take it. What if a computer program could help predict the patients at greatest risk of not taking their antidepressants?
A group of researchers at Harvard Medical School has developed software that they say can do just that, using information in the patient’s medical record. The study was published in Translational Psychiatry.
“Given enough time and adequate treatment, most patients with depression will get better,” said Roy Perlis, M.D., a professor of psychiatry at Harvard Medical School and senior author of this study. “While predicting the right medicine has received the hype, we wanted to ask a more mundane but clinically meaningful question: What’s the wrong medicine? Which patients are going to get their prescription and then never come back?”
To answer this question, Perlis and his team analyzed electronic health record data available from two large health systems in the Boston area. They looked for adult patients aged 18 to 80 who received a depression diagnosis and at least one prescription for one of nine common antidepressants (bupropion, citalopram, duloxetine, escitalopram, fluoxetine, mirtazapine, paroxetine, sertraline, or venlafaxine) between 2008 and 2014.
The final sample included 43,996 patients who had received at least one antidepressant and attended at least one follow-up visit 90 days or more after their first prescription. This patient sample included 70,121 total medication changes (for example, patients received a new prescription or switched medications). In total, 16,665 of these medication dispensations (23.77%) resulted in apparent treatment discontinuation, which as defined as a failure to follow-up on the prescription along with no evidence that the patient was seeking non-pharmacologic depression treatment.
In general, discontinuation rates were higher when a nonpsychiatrist prescribed the medication than when a psychiatrist prescribed the medication. Patients were also more likely to stop selective serotonin reuptake inhibitors (escitalopram, fluoxetine, paroxetine, and sertraline) compared with medications in other antidepressant classes. Among individual medications, venlafaxine had the lowest rates of discontinuation (20.78%) while paroxetine had the highest rates (27.71%).
Perlis and colleagues then used a machine learning algorithm to look for clues within the patient’s health records that might indicate a risk of dropout. The software looked exclusively at patients’ sociodemographic traits (age, gender, ethnicity) and medical history. To simplify the algorithm, medical history data was limited to procedural or diagnostic codes that appeared in at least 100 patients (3,582 total). Perlis explained that the researchers wanted to create a program that relied on information already included in patients’ medical records and not one that would require physicians to obtain additional information from patients during an office visit.
Overall, the software could predict which patients would stop taking their medication with about 69% accuracy. The accuracy varied when exploring individual medications; the model was best at predicting patients who would stop taking escitalopram and least accurate at predicting adherence to paroxetine. The prediction rates were similar between the two health systems, however, suggesting this program could be applicable across a range of clinical settings.
Perlis acknowledged his model is imperfect but said it could still provide value to physicians. “It can help us triage patients to make sure they stay in treatment,” he said.
For example, Perlis told Psychiatric News that patients who might be considered to be at particularly high risk of discontinuation based on their medical profile could be flagged to receive additional follow-up calls from the doctor or a mobile app that promotes medication adherence.
Additionally, since this model relies on basic patient medical data, there is much room for improvement, Perlis added. Currently, his team is looking at other easy-to-incorporate data that might be added, such as the patient’s genetic information or physician notes on the patient. They are also developing a dashboard to try and present the software findings to doctors in the most user-friendly way.
This study was funded by Oracle Labs, the Harvard Center of Research on Computation and Society, the Harvard Data Science Initiative, and the National Institute of Mental Health ■