Suicide Prediction Models May Lead to Greater Racial Health Disparities
Abstract
A study found that two suicide risk prediction models were less accurate for some underserved populations, highlighting the importance of auditing models to ensure they equally benefit people of all racial/ethnic backgrounds.
Models that predict patients most at risk of suicide may be less accurate at predicting this risk in people who identify as Black or American Indian/Alaska Native suggests a study published in JAMA Psychiatry.
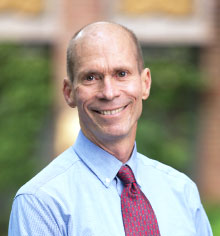
When implementing suicide risk prediction models, it is important to remember that a model that performs well on average may not perform well for everyone, says Gregory Simon, M.D., M.P.H.
“To us, one of the key messages of this study is: If you’re going to use tools like this, you have an obligation to look at the question of potential racial and ethnic biases,” said Gregory Simon, M.D., M.P.H., an investigator with Kaiser Permanente Washington Health Research Institute and a psychiatrist at Washington Permanente Medical Group.
Simon and his colleagues were aware of ongoing discussions around prediction models potentially perpetuating racial/ethnic disparities in other disciplines, such as criminal justice and education, which led them to investigate whether the same is true in health care settings. Because suicide risk prediction models rely on health records, they, too, may be a less accurate indicator for patients who experience disparities in access to health care, the authors wrote.
“We wanted to make sure any suicide risk prediction models introduced in health care settings reduce health disparities, rather than exacerbate them,” said the study’s lead author, R. Yates Coley, Ph.D., assistant investigator with Kaiser Permanente Washington Health Research Institute.
Simon, Coley, and colleagues gathered data on outpatient visits to a mental health specialist, including health record and insurance billing information, from seven health systems between January 1, 2009, and September 30, 2017. Suicide predictors used in the analysis included demographic characteristics (age, sex, race, ethnicity, and insurance type), comorbidities, mental and substance use diagnoses, dispensed psychiatric medications, prior suicide attempts, prior mental health encounters (including hospitalizations and emergency department visits), and Patient Health Questionnaire-9 (PHQ-9) responses. Patients reported their race/ethnicity during clinic visits. The analysis included nearly 14 million visits by 1.4 million patients; 768 suicide deaths were documented within 90 days of 3,143 visits.
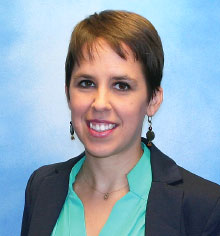
“All prediction models should be audited to see if they perform well across racial and ethnic groups, and if they don’t, health systems should pause before implementing them,” says R. Yates Coley, Ph.D.
The researchers ran two prediction models for suicide deaths that occurred within 90 days after an outpatient visit: a logistic regression model and a random forest model. Logistic regression is a standard, traditional statistical model that has been used in research for decades, Coley explained. It predicts the probability of a binary (that is, yes or no) outcome, such as whether a suicide death occurred in the 90 days following an outpatient mental health visit. Because the researchers included hundreds of potential suicide predictors, they used a selection process known as LASSO to identify the most prevalent predictors.
The random forest model is an example of machine learning, or artificial intelligence, Coley said. It explores the interactions between predictors and outcomes, such as the predictive relationship between prior suicide attempts and a suicide death depending on an individual’s race or ethnicity. Coley said her team expected the random forest model to be more accurate across races and ethnicities—but they found it was not.
The researchers used a measurement known as area under the curve (AUC) to determine each prediction model’s “discrimination,” which refers to the possibility that the model will apply a higher suicide risk score to a randomly selected visit that was followed by a suicide death within 90 days than another visit that was not followed by a suicide death, Coley explained. The higher the AUC, the better the model is at predicting suicides. An AUC of 50%, for example, is no better than flipping a coin, Coley said.
The AUCs were highest for White, Hispanic, and Asian patients. The logistic regression model had AUCs of about 82.8%, 85.5%, and 83.4% among White, Hispanic, and Asian patients, respectively. Random forest, meanwhile, had AUCs of 81.2%, 83.1%, and 88.2% among White, Hispanic, and Asian patients, respectively.
For Black patients, however, the logistic regression model AUC was 77.5%, while the AUC for random forest was about 78.6%. Among American Indian/Native Alaskan patients, the logistic regression and random forest model AUCs were only 59.9% and 64.2%, respectively. For patients with unrecorded race/ethnicity, the AUCs for logistic regression fell to 64.0%, while the AUC for random forest was 67.6%.
The authors hypothesized several reasons why suicide prediction models may be less accurate for some populations. Health record data may poorly predict suicide death because underrepresented racial/ethnic groups face higher barriers to affordable, culturally competent mental health care, or because suicide deaths among these populations may be misclassified as unintentional or accidental, or vice versa.
“There are various reasons why these kinds of problems could occur, and we cannot say exactly which reason is responsible,” Simon said. “It could reflect biases in health care delivery or differences in how people use health care. It may be due to too small a sample, and the solution could be to get more data on these populations. We may need better statistical methods to develop models that work for all people.”
“Suicide is the ultimate adverse event, and as psychiatrists it’s what we’re trained to prevent at all costs,” said Walter E. Wilson Jr., M.D., M.H.A., chair of APA’s Council on Minority Mental Health and Health Disparities. “It’s unfortunate to see another system fail certain populations that are already underprivileged and underserved.”
It is also vital to discover why the prediction models worked so poorly for individuals with no race or ethnicity recorded in the study, Wilson said. “Is this a population that is distrustful of the health care systems, or are these individuals for whom access to care is so scant that they don’t have as many encounters during which this information is recorded in their health record data?”
The authors also hypothesized that suicide risk prediction models are less accurate for some groups because practitioner bias and institutionalized discrimination may lower the likelihood that underrepresented populations will receive a mental health diagnosis or treatment. This is an important theory to investigate, Wilson said.
“We all bring biases into the office because we’re human, but biases that cause us to treat patients differently prevent us from identifying important clinical behaviors that may be suicide risks,” he said. “It is vitally important to be aware of practitioner biases, understand them, and not be afraid to address them.”
The study was funded by grants from the Mental Health Research Network from the National Institute of Mental Health. ■
“Racial/Ethnic Disparities in the Performance of Prediction Models for Death by Suicide After Mental Health Visits” is posted here.